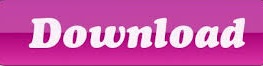
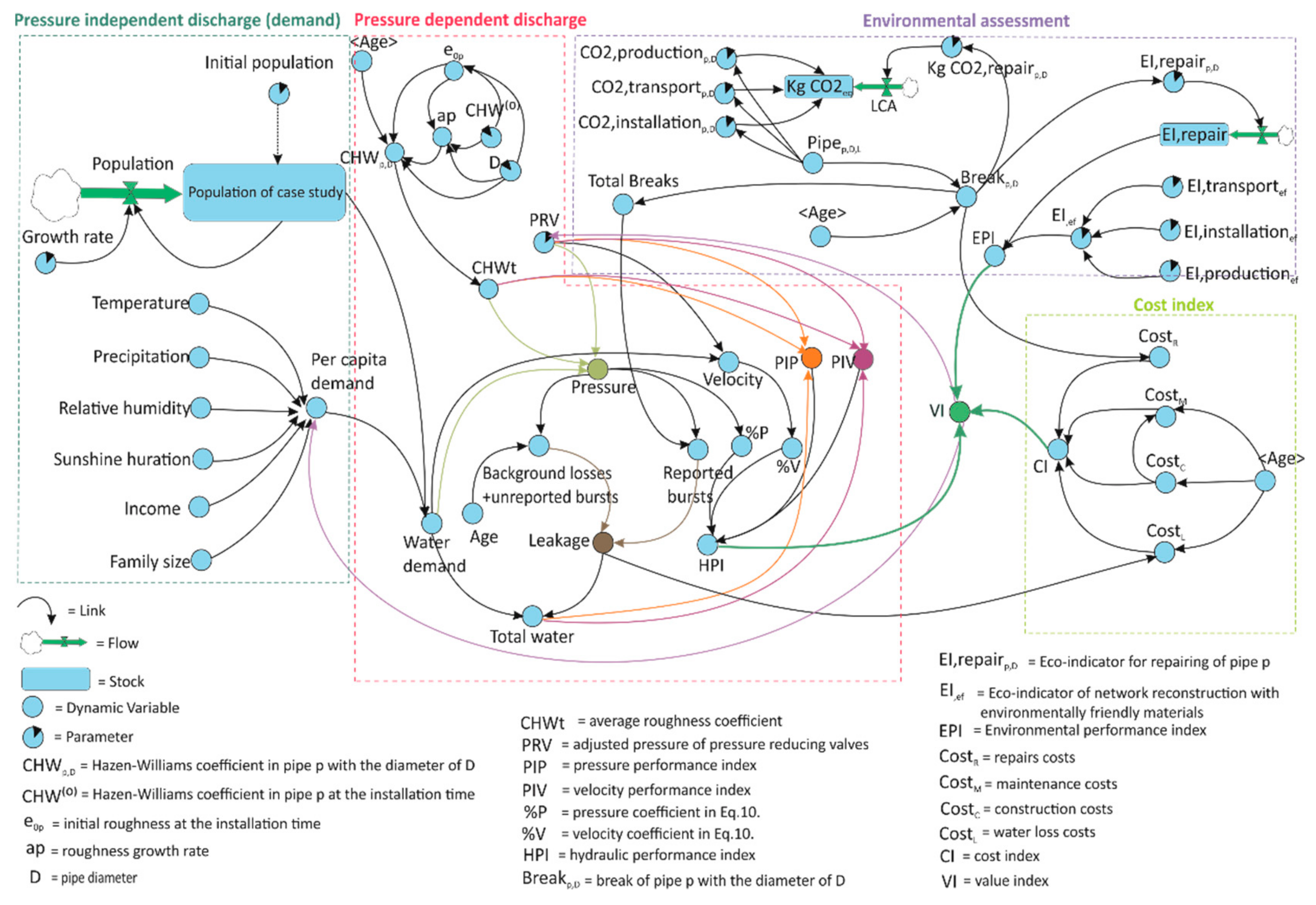
Indeed, estimating dwell times as fixed values, ideally the same for all runs and all stations, can result in deviations between actual and planned operations, with a subsequent deterioration in system performance. By contrast, estimation of dwell times on the basis of flows becomes fundamental in the planning phase. Due consideration of this factor is fundamental because, if it were to be repeated, it would make a further contribution to passenger discontent. Specifically, the former takes account of the possibility that not all passengers can board the first arriving train, but only part of them, due to overcrowding, with a consequent increase in waiting times. First and foremost, it leads to introducing elements such as convoy capacity constraints and the assessment of dwell times as flow-dependent factors which make the simulation as close as possible to reality. In particular, considering travel demand within the analytical framework presents a two-sided effect. Therefore, after an overview of the main rail simulation and optimisation models proposed in the literature, the paper focuses on the main issue concerning interactions of rail service with travel demand flows and the energy domain.įirst, as rail transport, just like any other transport system, has the task of moving people or goods around, a realistic and accurate cost–benefit analysis cannot ignore the flow features involved.

However, a rail service is generally considered as a stand-alone system, and other systems, which are strictly connected with it by means of reciprocal interactions, are regarded just as constant input variables or, at worst, totally neglected. Hence developing appropriate techniques and decision support tools for optimising rail system management, both under ordinary and under disrupted conditions, would clearly affect the modal split in favour of public transport and therefore bring about a considerable reduction in the externalities caused by the use of private transport, such as air and noise pollution, traffic congestion and accidents, conferring clear quality-of-life benefits for both transport users and non-users (i.e. Thus, re-establishing ordinary operational conditions may require excessive amounts of time and, as a consequence, an inevitable increase in discomfort (user generalised cost) for passengers, who might decide to abandon the system or, if already on board, exclude the railway system from their future choice set. systems which are not integrated into an effective network) or when a breakdown occurs on open tracks.
:max_bytes(150000):strip_icc()/IntroductiontoSupplyandDemand1_2-578635efad694c929704cbaf6884fb55.png)
However, it is highly vulnerable in the event of breakdowns: a faulty convoy cannot be easily overtaken and sometimes cannot be easily removed from the line, especially in the case of isolated systems (i.e. Indeed, rail transport is environmentally friendly (low pollutant emissions), high-performing (high travel speeds and low headways) and competitive (low unit costs per seat-km or passenger-km), while presenting a high degree of adaptability to intermodality. Rail transport benefits from several specific features which make it a key element in public transport management, above all in high-density contexts. Finally, in an attempt to provide a comprehensive framework an overview of the main metaheuristic resolution techniques used in the planning and management phases is shown.
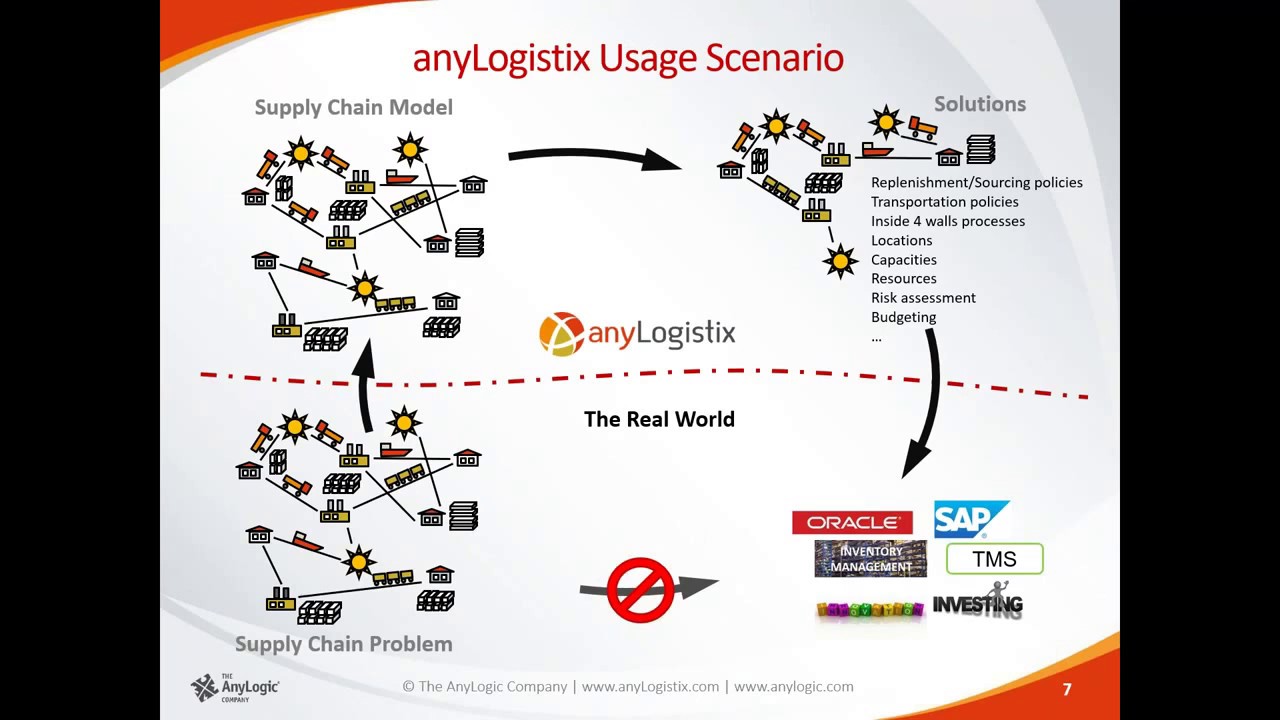
The main issues concerning the interaction of rail services with travel demand flows and the energy domain are also described. By contrast, within rail optimisation models, both planning (timetabling) and management (rescheduling) phases are discussed. Rail simulation models are classified according to the level of detail implemented (microscopic, mesoscopic and macroscopic), the variables involved (deterministic and stochastic) and the processing techniques adopted (synchronous and asynchronous). For this purpose, an extensive overview of the rail simulation and optimisation models proposed in the literature is first provided. Given our underlying belief that to build a robust simulation environment a rail service cannot be considered an isolated system, also the connected systems, which influence and, in turn, are influenced by such services, must be properly modelled. The paper aims to provide an overview of the key factors to consider when performing reliable modelling of rail services.
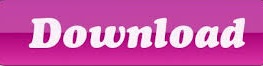